Volume 24: The 2018 Election, Who Projected It Best?

A log-loss comparative analysis of quantitative and qualitative 2018 U.S. House of Representatives election projections
“Well, how did your projections do?” – Dale Cohodes.
It will come as a shock to nobody that I maintained a personal set of projections for the recently completed elections to the House of Representatives. It may surprise you more to know that reviewing my projections alongside the so-called “professionals” gives us an excellent opportunity to think through one of our favorite topics—probability. Elections are an interesting class of random event: probabilistic with a single trial and a discrete outcome. The tools we have to predict their outcome—polls, demographics, past voting patterns—result in distributions that include deviations from a mean. But no matter how much we’d like to, we cannot re-run the recent election in Georgia’s 7th or North Carolina’s 9th Congressional district, even though each was decided by fewer than 1,000 votes. And no matter how small the margin, the candidate with a plurality of the votes wins; a margin of 10,000 votes or 1,000,000 votes results in the same practical outcome. Elections are fundamentally different from random processes like flipping a coin or tomorrow’s high temperature.
Because of this, a simple question about forecast quality can be extended to provide insight into the general nature of probabilistic forecasts.
• What’s a good probabilistic forecast? • Whose House projections were the best?
What’s a good probabilistic forecast?
Let’s start with the basics. We define a probabilistic forecast as a statement of the likelihood of the occurrence of a discrete event, made by a person (the forecaster) before the event is decided.(1) When a sports handicapper says the Wolverines have a 75% chance of winning their next game, that is a probabilistic forecast. When your local weatherman says there is a 50% chance of rain tomorrow, that is also a probabilistic forecast.(2)
We already know that probabilistic thinking is a skill the human mind does not necessarily possess. We are not good at translating concepts like “possible,” “likely,” and “almost certain” into quantitative likelihoods of occurrence. If we are told that the probability of something happening is 80%, and it doesn’t occur, we are frequently quite distraught. And maybe we should be. But a forecaster who places an 80% probability on an event that always happens is also not doing a very good job. Saying that there is an 80% chance of the sun rising tomorrow is not a show of forecasting skill, but rather a lack thereof.
So how do we know a good probabilistic forecast when we see one? Consider a weatherman(3) who says that there is a 50% chance of rain on Tuesday. If it rains, then the weatherman wasn’t wrong; it was clearly something in the realm of possibilities. But the rub is that, if the same prediction is made the following day, and the sun in fact comes out, the forecast is equally good—and equally bad. Over the two-day span, the forecasts did not add any informational value. A weather forecast that says day after day after day that the chance of rain is 50% is useless. Such a weatherman would soon be exited from your local television station, and they should be.
But let’s move to Phoenix, where it rains only 10% of the time on average.(4) Now a forecast showing a 50% chance of rain that is borne out is a fantastic one. On the other hand, if it doesn’t rain, then it isn’t such a bad prediction, as it almost never rains in Phoenix. A 2-day forecast showing a 50% chance of rain each day, one day of which is borne out, has a lot of value in the desert. Which brings us to a principle: the quality of a forecast depends on how different it is from the probability that would be assigned in its absence.(5) Showing a few different sets of Phoenix predictions gives us more information on which weathermen should keep their jobs.

First, let’s check against our prior. It rains in Phoenix 10% of the time, and we had one shower in ten days. Check; our expectations for long-run rain held out.(6)
Let’s start with Weatherman Ugly. These were some bad predictions. Not only did he think rain was likely on five dry days, but he also put a probability of 0% on the one day where it did rain.(7) This man is bad at his job; listening to him is literally worse than just going with the long-term average of 10% chance of rain every day.
Which is precisely what our Bad Weatherman did. These predictions were not so bad as his Uglier brother-in-forecasting, but they are also essentially useless. You don’t need a degree in meteorology or fancy weather radar to make these predictions. He should still be fired.
On the other hand, our Good Weatherman in fact did some strong work. It rained on one of the three days on which he thought it might rain; 33% realization on a 40% prediction isn’t bad. He also confidently predicted no rain on seven days and was correct on each. Using these predictions is far superior to simply relying on the long-run average.
Before we finally describe our metric for the quality of a probabilistic forecast,(8) let’s run through one more set of forecasters. For this, we go back to a wet sub-tropical climate where we can expect rain 50% of the time.

Our Bad Forecaster…well he’s still doing his thing, going with the historical average. I’m getting tired of this guy. Our Good Weatherman puts up a reasonable showing. When he predicts a 60% chance of rain, it rains; when he predicts a 40% chance of rain, it doesn’t. It almost seems that he is better at this job than he thinks he is. The days are segregated properly, but he lacks confidence. And this is made our Better forecaster better. Even though each individual day is still far from certain, these predictions are clearly better than the previous set. Perhaps these predictions should also be more confident; after all, on days where rain was likely, she was right 100% of the time, not 80%. But we’ve come far enough to state two principles of probabilistic forecasting:
A probabilistic forecast is “good” if it is better than a relevant, uninformed estimate.
A more certain forecast is better than a less certain forecastif it is correct.
Now for our very simple metric of the quality of a probabilistic forecast: log-loss.(9) For a probabilistic forecast with probability p,
If the event occurs,
Log-loss = -1 * log ( p )
If the event does not occur,
Log-loss = -1 * log ( 1 – p )
That’s it. Just be sure that for your “log,” you use the natural log of base e. Also, don’t try to use a probability of 0% of 100%; use a very small number of your choice.(10) Rather than describe what this looks like, let’s visualize it:

The first thing that we see is that log-loss is a penalty. See those big numbers for low probability events that occur (and high probability events that don’t)? You don’t want to be out there. Don’t make especially confident predictions that don’t come true. The two lines intersect at a probability of exactly 50%. Estimating a 50% probability on a coin flip is equally good or bad no matter what you forecast, or what happens.
Log-loss is especially useful when you sum it over several probabilistic forecasts.(11) We can do so for the first set of weather forecasters we considered earlier.

As we expected, our Good Forecaster did the best. One drawback of log-loss is that the number has little objective meaning. Our Good Weatherman had a total log-loss of 1.945, but that means nothing on its own. However, when we compare multiple sets of forecasts,(12) we can start to have some qualitative and quantitative opinions. Our Good Forecaster is much better than our Bad Forecaster, and Ugly is way off base.
Whose House projections were the best?
And now we apply what we’ve learned.
Elections to the United States House of Representatives are a dream for forecasters and statisticians alike. A large natural experiment, with 435 simultaneous trials, quantitative results, a large data set, local specifics to learn, and many other things that just can’t be quantified; each of these was present on November 6, 2018.
Even forgetting those running for office, managing the two political parties, and spending the money, there was a lot of interest in the results of these elections. Therefore, many people (and groups) attempted to forecast the results. Not only does forecasting provide a public service of some value, it also provides a lot of hits on one’s website.
Broadly speaking, there are two types of election forecasters. Quantitative forecasters look for publicly available information like polls and fundraising, as well as endemic variables like economic conditions. Using some type of fitting, they decide which of these variables are predictive of upcoming House elections results. They also attempt to determine the best way to “mix” these variables together to predict results. Because of the nature of their forecasting, they typically offer numerical, probabilistic predictions: Candidate A has a 75% chance of winning. They might also predict vote shares: Candidate B is projected to win 45% of the total 2-party vote.
Qualitative forecasters use the same variables but add in other factors, such as knowledge of the candidate or the district that isn’t quantifiable. Typically, they offer qualitative forecasts; for example, “Candidate C is likely to win the election”. I created my own set of qualitative forecasts.
If you didn’t skip the first section, you are probably getting excited, because this is the perfect setup for a log-loss analysis. The only slight hitch is that we are forced to change the qualitative rankings into probabilities. I used the following mapping, expressed as the probability of the Democrat winning the given seat:(13)
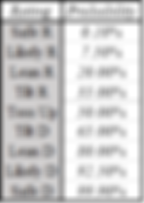
Figure 1 - Race Rating to Probabilities
Recall that the log-loss analysis runs into trouble with probabilities of 0% and 100%, hence the small deviations for Safe predictions.
As a first cut of the data, we can look at each forecaster to see their distribution of projections. (See the appendix for descriptions of the professional forecasters shown in Figure 2.) Note that only Inside Elections and I used the “Lean” designation. Sabato’s Crystal Ball took a stand just before the election, forbidding the use of the “Toss Up” designation.(14)

Figure 2 - Seat Projection Distributions
We can already glean a few interesting pieces of data. IE and CNN (and RCP to a lesser extent) had many more seats viewed as Safe R; other forecasters took seriously the polls showing at least the possibility of a truly massive Democratic wave. Similarly, RCP had very few Safe D seats, while Crosstab, 538, and I had many. I’m not quite sure why RCP considered the result in New York’s 18th district or Iowa’s 2nd to be in doubt; this hurt their performance. Like the quantitative forecasters, I was not afraid to call some seats previously held by Republicans as “Safe D.” For example, there was no doubt in my mind that Democrats would win two seats in Pennsylvania that had changed due to redistricting. Also, Sabato’s proscription of the Toss Up rating greatly increased the number of Lean D seats in his projection.
Using this raw data, as well as the probabilities in Figure 1, we can translate into a projected number of Democratic seats won for each forecaster.(15) We can also see the number of seats in which each party was favored by a forecaster. I call the former metric the Mean and the latter the Median.(16)

Figure 3 - Projected Seats Won
The totals won fell within a reasonably tight range: 7 on median and 9 on mean. It bears noting that each forecaster predicted a skewed distribution, with the mean Democratic seats won greater than the median. This is again due to the potential wipeout of the GOP House caucus; evidence of this possibility was seen by both the qualitative and quantitative forecasters. A GOP enthusiasm plunge could have put normally safe seats at risk. Quantitatively, there is also a tradeoff of the aggressive gerrymanders created by the GOP state governments in 2010. A greater likelihood of gaining a majority in the House is balanced somewhat by the potential of losing more seats than expected in extreme scenarios.(17)
With the description out of the way, we can look and see how everybody did. Remember that log-loss analysis is like golf: a lower score is better.

Figure 4 - Log-Loss Results
And there it is: Larry Sabato’s team at the University of Virginia emerges victorious, with some room to spare. 538 is the runner-up, and my projections pull in a solid bronze. Referencing Figure 1 again, it is interesting that our winner was the only contestant that made a call on every race; fortune truly favors the bold. I was happy with my result against the professionals, but it is important to remember that I had a key advantage. Not only could I use all the data that they used, but I could—and did—use their ratings and commentary. Without this, I could not have been competitive.
That said, it is interesting to consider a few contests specifically. For each of the 435 seats I calculated the average log-loss for all forecasters. Comparing each forecasters individual log-loss to this average is a measure of the relative quality of their forecast for a given seat. A “Safe” prediction is easy to make when it agrees with everybody else. A correct “Likely” call when everybody else was only Leaning adds value.
My best call was in Oklahoma’s 5th district, where incumbent Republican Steve Russell was considered Safe by three forecasters. Fortunately for Democrat Kendra Horn, he was not safe, in what was probably the biggest upset of the night. My “Likely R” rating was a good one. I’d seen a lot of Democratic strength in local Oklahoma elections. I also thought that Democratic strength in upscale and educated suburbs might extend to Oklahoma City. Because of the large penalty for incorrectly calling races Safe, this seat was the most critical of the night forecasters to get right.
I also made a good call in New York’s 11th district. I don’t live in this district, but its border is only a few miles from my home. I’ve also met with Congressman-elect Max Rose, know people who worked for his campaign, and saw him break through the crowded NYC airwaves with a ton of positive coverage. I rated this one Tilt R, while every other forecaster said Lean R. Two weeks before the election, most predicted Likely R. Local knowledge seemed to help me in other places as well; two competitive Michigan districts, both located near where I grew up, were also among my best calls (Tilt D in Michigan 8th; Likely D in Michigan 11th).
Of course, I was far from perfect. I didn’t think Lucy McBath was likely to win the Georgia 6th, site of a close Democratic loss in a special election in 2017. I had this one Lean R. To make matters worse, I thought Republican Rob Woodall in the neighboring Georgia 7th was at real risk. Despite my Toss Up rating, Congressman Woodall snuck back into Congress in one of the night’s closest races.
Outside of Metro Atlanta, I also fared poorly in upstate New York. I didn’t expect this to be an area of significant Democratic strength, keeping the 19th and 22nd districts as pure Toss Ups. To make matters worse, I thought Republican incumbents were at risk in the 21st (Stefanik, Likely R), 23rd (Reed, Likely R), 24th (Katko, Lean R) and 27th (Collins, Lean R). Even with my call in the 11th, I barely broke even in the Empire State despite living here.
South Carolina’s 1st district, another surprise on election night, bears mentioning. This district, a conservative one represented by former Governor Mark Sanford of Appalachian Trail fame,(18) was not ready to accept unapologetic Trumpist Katie Arrington. Even Crosstab, the qualitative forecaster at the very low end of Safe R seats, did not see this one coming. California’s 21st, which Democrat TJ Cox stormed back to win as California conducted its usual deliberate count, was also home of a wide split in opinion. I fell on the wrong side, thinking David Valadao would hang on (Likely R). Iowa’s 3rd district, previously held by noted non-entity David Young,(19) seemed like an easier pickup to me (Lean D) than New Jersey’s 7th, featuring the popular incumbent Leonard Lance (Tilt D). I also didn’t get ahead of myself in Illinois, keeping the 13th and 14th districts at Lean R. Democrat Lauren Underwood won the latter.
While I could go on for this whole article listing districts,(20) this is as good a stopping point as I’m likely to find. Should you be interested in knowing more, all the picks are available in a spreadsheet, which is linked in the appendix.
Elections are important for more than simply log-loss analyses of their forecasters. You may be interested in my opinions about this one’s winners, losers and what it all means.
Our views on elections are shaped, partially, by the coverage on election night. This coverage, in turn, is improperly shaped by the order in which states report election results. On election night 2018, an early cable news message set in that the night was not going to be a good one for Democrats based on early returns in Kentucky, Indiana, and Florida. With this message set in, many think that the election was a close one; after all, Democrats won the House and Republicans the Senate, an effective tie?
Like many things you see on television, this is not really true. Democrats won the national vote in the House by almost 9%. The Senate, where the GOP claimed a great victory? Democrats won the vote there by about 20%.(21) This is the largest margin of victory in any midterm, by either party, since the Democratic victory in 1974 during the Watergate aftermath.
Also pierced forever is the idea of Donald Trump as a person with some magical political skill. He spent the month before the election traveling the country, holding rallies, attempting to make the election about him. Unfortunately, the candidates he stumped for did not perform especially well. All those trips to West Virginia did nothing to help Patrick Morrisey. Big attendance in Big Sky Montana didn’t prevent Democrat Jon Tester from gaining a comfortable victory. Thousands of Republicans showed up for a Trump rally in Elko, Nevada, with Senator Dean Heller. Democrats skipped the show and swamped the polls. Trump stumped for winning candidates as well, but there is no evidence that candidates that he stumped for outperformed.
Democrats had disappointments as well. Rick Scott and Ron Desantis will represent Florida in the Senate and the state house. Beto O’Rourke’s tens of millions left him 3% short of defeating Ted Cruz. Claire McCaskill in Missouri and Joe Donnally in Indiana couldn’t overcome the increasing red hue of their respective states. But America spoke in a voice that was as clear as she ever uses in an election. That voice was, at all levels, a broad and deep rejection of Donald Trump and Trumpism.
Appendix
Congratulations on making it to the very first LobbySeven Commentary appendix. It amazes me that this is the first time I’ve broached such sacred ground.
First, my spreadsheet is available here for you to play around with. I do not promise to maintain it; if you do find an error, they happen, and pointing it out would be appreciated.
As discussed, I used the projections of seven “professional” forecasters and my own. The pros are:
CNN (Harry Enten), whose predictions I am not available to find active on their website, but they are available here.
I have a few others that I would have included but they didn’t provide the data in any reasonable format. But they still did good work! And if you will type it all out in Excel format, I’ll add them in.
How do you know that my projections were done before the election, rather than backfilled? Well, I now wish I’d posted them before, as a record, but you’ll just have to trust that this would be a lot of work to pretend that I’d falsified a set of projections that, frankly, were barely middle of the road.
As mentioned, one of the choices I was forced to make was a translation between qualitative race ratings (i.e., Lean, Likely) and probabilities. Some of the forecasters listed their probabilities; I didn’t use them, putting everybody on the same scale. Maybe this was a mistake, but it was easier to code and I liked the consistency. For the two quantitative forecasters (538 and Crosstab) I ran two sets of projections. In the first, I “bucketed” their probabilities into the relevant qualitative rating, and then used the “consistent” scale. I did this because they have a lot of races at probabilities of 98% or 99% toward the favorite, and I didn’t want them to be negatively impacted by the small divergence from safe. In fact it had the opposite effect; both of the quantitative forecasters did better if you use their straight probabilities. I’ve used only these in this paper, but both sets are in the spreadsheet.
At the time of this writing, there are only one House seat has not been decided: the North Carolina 9th. It appears that there was some type of massive election fraud in the absentee ballots in this district. It was allegedly conducted by an operative aligned with the Republican candidate, and that something similar happened when the GOP incumbent was defeated in the primary. The state board of elections made the bipartisan, unanimous decision to not certify the election results. I have no idea what will happen here; there could be a new election ordered, or the House might refuse to seat Republican Mark Harris (who benefitted from the skullduggery). The race is an exceptionally close one no matter what; the uncertified vote differential is about 900. I am not including it in the log-loss analysis; forecasters were not really thinking about this type of situation when placing their bets. That being said, if it fell D, Sabato would come out even further ahead.
My sheet therefore has a Congress of 235 Democratic seats, 199 GOP, and 1 ¯\_(ツ)_/¯.
I think from here you can follow the remainder of my work, and I hope you do.
-NC
(1) We are going to stay mostly in the realm of events that have only two possible outcomes, such as elections in the United States or getting in a car accident on your way home. You can have probabilistic forecasts that are not binary, like a soccer game, which frequently ends in a tie. There are also predictions that are not probabilistic. For example, a single-value forecast, like tomorrow’s high temperature, is not probabilistic.
(2) Sports gambling and weather forecasting are by far the two most commonly used examples of probabilistic forecasting.
(3) I try to write gender-neutrally, but “weatherperson” just doesn’t work. I’ll make it up to you by describing later how many women were newly elected to Congress.
(4) All weather statistics are fictitious, made up by me.
(5) I’m avoiding Bayesian language to the extent possible, but this all translates. The informational value of a probabilistic forecast is proportional to the extent that it differs from your Bayesian prior.
(6) Of course, ten days is too small a sample size to test a prior as strong as long-run average weather patterns. It is eminently possible to flip a coin ten times and get ten heads. You can also roll a fair die ten times and get five 3s.
(7) We all know that we shouldn’t ever be putting probabilities of literally 0% or 100% on any prediction. I did so for simplicity here; feel free to assume your own arbitrarily small number.
(8) I can sense your excitement from here.
(9) The version I’m showing is for a binary prediction. There is a simple extension for predictions with multiple possible states, but this is enough math. Get to the election forecasts already!
(10) I’m using 0.001 or 0.1% as my arbitrarily small number for log-loss calculations. We math people call this an epsilon.
(11) Like 435 elections for the U.S. House of Representatives.
(12) Such as several different forecasters predicting the results of said elections.
(13) The analysis is robust for small changes in these probabilities, as well as small changes in epsilon.
(14) For the quantitative forecasters, I translated them back to ratings based on the same key used in Figure 1.
(15) As a reminder, a party needs 218 House seats to have a majority.
(16) Strictly speaking, this is not a median, but it lent a nice symmetry to the verbiage. Toss Ups counted as 0.5 for each side, in case you were wondering.
(17) It depends on assumptions, but in the current maps the Democrats would be expected to win excess seats if they won the national House vote by around 10%.
(18) Look him up if you don’t know about this.
(19) Not to be confused with Don Young of Alaska, who faced down a strong challenge to win his seat for the 24th time.
(20) Factcheck: true.
(21) Yes, this is skewed by California running only two Democrats in the election. But even correcting for this, the national Democratic victory in the Senate would still be around 12%. And if the GOP’s strongest case for their electoral success is that the numbers looked bad because they couldn’t get enough votes to get a candidate into the Senate race in our nation’s most populous state, then they have officially lost the argument.